Revolutionizing Patient Care: Artificial Intelligence empowering Digital Medicine
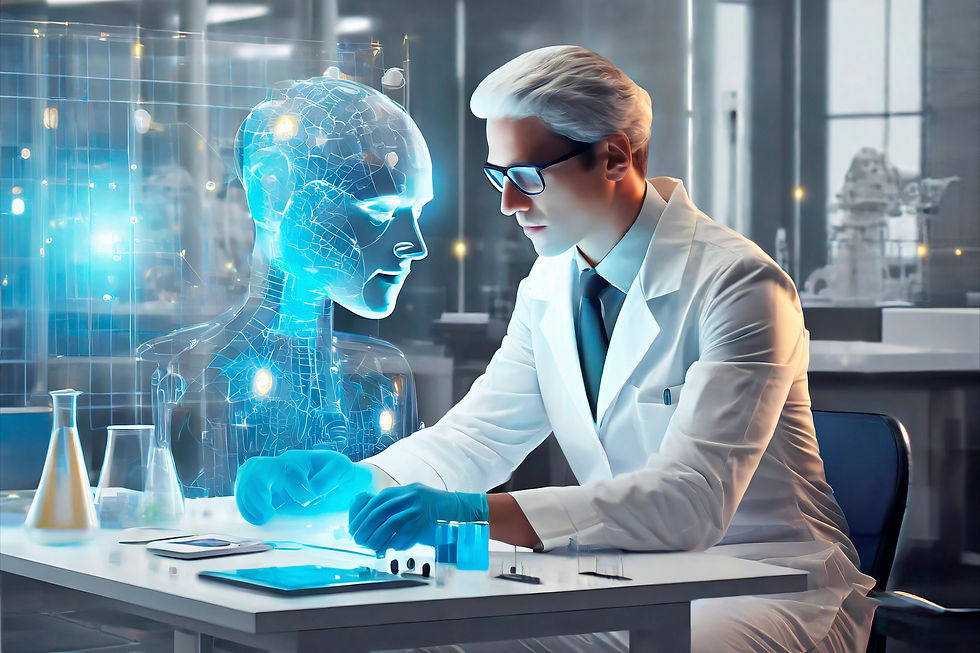
Blog by: Dr. Suhail Chughtai, FRCS, FFLM
Artificial Intelligence (AI) is rapidly reshaping the healthcare landscape, particularly in diagnostics, personalized treatment plans, and patient outcomes. AI-powered tools are transforming clinical practices and improving healthcare efficiency, accuracy, and accessibility. This article delves into how AI is revolutionising patient care and the challenges faced in its deployment and provides a glimpse into its future impact on healthcare.
AI IN DIAGNOSTICS: IMPROVING ACCURACY AND SPEED
AI tools, particularly machine learning (ML) and deep learning (DL), have shown remarkable capabilities in diagnostic imaging, pathology, and radiology. These tools can process vast amounts of data quickly and identify patterns that might be missed by human clinicians.
Medical Imaging
AI algorithms, such as convolutional neural networks (CNNs), are being utilised in radiology to detect diseases like cancer, fractures, and neurological disorders. For instance, Google's AI algorithm has demonstrated superior accuracy in detecting breast cancer on mammograms, often outperforming radiologists (McKinney et al., 2020).
Pathology
AI tools analyse tissue samples and detect diseases like cancer at a microscopic level. Studies have shown that AI can predict the spread of cancer with a level of accuracy comparable to pathologists (Cireşan et al., 2013). These advances not only speed up the diagnostic process but also reduce human error, leading to early disease detection and improved patient outcomes.
PERSONALISING TREATMENT PLANS: TAILORED HEALTHCARE FOR BETTER OUTCOMES
AI is enhancing personalised medicine by using patient-specific data, including genetics, lifestyle, and medical history, to create customised treatment plans.
Precision Medicine
AI models help in predicting how a patient will respond to specific treatments based on their genetic profile. For instance, IBM’s Watson for Oncology analyses clinical trial data and literature to recommend personalised cancer treatments (Joustra et al., 2018).
Predictive Analytics
AI tools are also being used to forecast potential health issues before they arise. By analysing patterns in patient data, AI can suggest preventative treatments or lifestyle changes, thereby reducing the risk of chronic conditions like diabetes and heart disease. These AI-driven approaches allow for more precise and individualised treatments, leading to better patient satisfaction and long-term health outcomes.
TRANSFORMING PATIENT OUTCOMES: THE ROLE OF AI IN MONITORING AND FEEDBACK
AI is revolutionising patient outcomes by improving the monitoring of chronic conditions and enhancing communication between patients and healthcare providers.
Wearable and Remote Monitoring
AI-powered wearables continuously collect and analyse health data. These devices can alert patients and doctors about critical changes in real time, such as abnormal heart rates or glucose levels. For example, AI-enabled smartwatches can predict potential heart attacks by analysing heart rhythm patterns (Tison et al., 2018).
Telemedicine
AI tools also optimise telemedicine platforms, enabling healthcare providers to offer personalised care remotely. This becomes particularly important in rural and underserved areas, where access to specialists is limited. By continuously analysing patient data and providing immediate feedback, AI enhances patient engagement and ensures timely interventions.
DEPLOYMENT METHODOLOGY AND CHALLENGES
Data Quality and Privacy
AI models rely heavily on large datasets to train algorithms. However, inconsistent or biased data can lead to inaccurate results. Furthermore, patient data privacy remains a major concern, especially under regulations like GDPR in the UK.
Integration with Existing Systems
Integrating AI tools into existing healthcare infrastructure can be complex. Hospitals and clinics may need significant upgrades in their electronic health record (EHR) systems to accommodate AI-driven solutions.
Trust and Adoption
Clinicians and patients must trust AI tools for them to be effective. There is often resistance to adopting AI, with some fearing that it will replace human expertise. Addressing these issues requires robust data management strategies, compliance with regulatory standards, and comprehensive training for healthcare providers.
CASE STUDIES SUPPORTING AI IN HEALTHCARE
DeepMind's AI for Eye Disease
DeepMind’s AI system has been used to diagnose over 50 eye diseases with human-level accuracy. In one trial, it accurately detected diabetic retinopathy, a leading cause of blindness, in 94% of cases (Gulshan et al., 2016).
AI in Orthopaedics
In orthopaedics, AI tools help surgeons with preoperative planning and intraoperative guidance. For example, AI-powered navigation systems are being used in joint replacement surgeries to improve precision and reduce complications.
FUTURE VISION: AI’S POTENTIAL IN HEALTHCARE
AI-Driven Drug Discovery
AI will play a pivotal role in speeding up the drug development process, identifying potential drug candidates in less time and at a lower cost (Ralph et al., 2019).
Advanced Robotics
Robotic systems powered by AI will assist in surgery, providing more precision and enabling minimally invasive procedures, ultimately reducing recovery time for patients.
AI as a Healthcare Companion
AI will not only assist healthcare professionals but will evolve into virtual healthcare assistants capable of offering real-time, evidence-based advice to patients, improving overall patient care management.
CONCLUSION
Artificial Intelligence is undeniably transforming the healthcare landscape. By improving diagnostics, personalizing treatments, and enhancing patient outcomes, AI is poised to revolutionise the way healthcare is delivered. While challenges in deployment exist, the future of AI in healthcare holds immense promise for improved patient care, efficiency, and cost-effectiveness.
DISCLAIMER
The content presented in this publication includes references, insights, and excerpts derived from external sources and authors. Every effort has been made to credit the original authors and sources appropriately. If any oversight or misrepresentation is identified, it is unintentional, and we welcome corrections to ensure proper attribution. The inclusion of external materials does not imply endorsement or affiliation with the original authors or publishers. This publication is intended for informational and educational purposes only, and the views expressed are those of the author(s) and do not necessarily reflect the opinions of the referenced sources.
Comments